Unlocking Organizational Value: The Transformative Power of AI in Knowledge Management
- Patricia Liden Kutxfara
- Nov 15, 2023
- 7 min read
Updated: Apr 5, 2024
Last week, I initiated a dialogue on Knowledge Management, a topic that has always captured my attention and inspired me to embark on this evolutionary journey of ideas. My focus is on revisiting concepts from eminent thinkers of our era and exploring how Artificial Intelligence can be contrasted with and integrated into these principles. I started reflecting on traditional knowledge management, addressing the dependence of organizations on the knowledge and experience of their employees, and the challenges faced in retaining and sharing this knowledge.
Just remembering that the goal of this journey is to deepen the understanding of how artificial intelligence, applied in knowledge management, can fill still persistent gaps in companies, especially regarding the retention, sharing of knowledge, and the monetization of the intellectual capital generated by business operations, as well as demystifying the much-feared issue of AI taking over 100% of human work. I invite you to be with me in this journey!
Today, I advance a bit more in the theory of Nonaka and Takeuchi, known as the Knowledge Spiral, sketching a comparison with AI.
I remember when I did my first MBA at the Pontifical Catholic University of São Paulo, BR, twenty years ago, and this book was given to us as part of the course curriculum. It was then that I first encountered the topic of Knowledge Management. I won't say it changed my life, because the discipline that did that was Project Management, but Knowledge Management and Organizational Change Management also fascinated me, and to this day, I work with them in conjunction with Project Management and People Management.
The Knowledge Spiral offers a valuable perspective on how information evolves into tacit and explicit knowledge, emphasizing the importance of collaboration and knowledge creation, which, let's face it, is the greatest asset of companies today and the essence of all artificial intelligence.
Next, I will explore this theory in detail. But first, as I mentioned last week, I will start by conceptualizing according to these authors:
Tacit knowledge – is that which is not easily expressed in words or formalized. It's a type of knowledge that resides in personal experiences, intuitions, practical skills, and individual perspectives. It can include things like practical experience gained over the years, an expert's intuition in solving complex problems, or for example the motor skills of a craftsman.
Explicit knowledge – compared to tacit, is more objective and can be easily communicated through language, written documents, graphs, and other forms of formal and tangible communication. This is the type of knowledge that can be easily transmitted in training manuals, technical documents, books, blogs like this one of mine, and so on.
Transforming Tacit into Explicit for Organizational Innovation
Nonaka and Takeuchi emphasize that transforming tacit knowledge into explicit knowledge is crucial for innovation and organizational development. They argue that effective organizations are those that can create an environment facilitating the conversion of tacit to explicit knowledge, thereby promoting continuous learning and innovation. This process is fundamental for the growth and adaptability of organizations in an ever-changing environment.
What is the biggest challenge companies face today in their digital transformation?
Here's the hook... from this perspective, AI can assist organizations in solving problems related to the retention and management of knowledge generated in their environment, managing their intellectual capital more intelligently and thus monetizing it, through the implementation of AI agents.
Remember last week's challenges that I've shared?
Clarifying that usually AI operates on explicit knowledge, which is stored in the company's databases in structured format (data resulting from their business processes or purchased data, why not?) and unstructured format (documents, spreadsheets, presentations, data from their operations on social networks, platforms like Miro, Jira, for example, and so on).
However, with the introduction of large language models (LLM) like GPT-3 or GPT-4, in the interaction between company employees and AI systems, there is a growing opportunity to also capture and formalize tacit knowledge. The human interaction with the machine allows it to learn and absorb the experience, personal perspectives, and knowledge of those who operate it.
These systems are designed to learn from large volumes of text, and when interacting with people, they can absorb additional information and nuances that reflect the users' experience and knowledge.
When people interact with AI systems (agents), like chatbots or virtual assistants, and provide feedback, corrections, or contextual information, this can help refine the system's performance. The machine can learn from previous conversations and experiences and improve its responses and understanding.
This bidirectional interaction between humans and machines is one of the ways AI can be adaptive and continuously improved.
In this way, the management of organizational knowledge truly establishes itself and innovates to generate absolute value compared to what was previously intangible value, as AI allows knowledge, which was previously only in the employees' heads, to be more accessible, applicable, and adaptable, making it a strategic resource to drive performance and competitiveness of companies.
And not least importantly, it becomes a helpful tool for company employees to easily access shared knowledge from other colleagues, who, for example, might be on the other side of the world. Without AI, they would not have access to this knowledge. Or not so fast, or not so full of value.
Understanding the theory to make sense
The Knowledge Spiral is a theory developed by Ikujiro Nonaka and Hirotaka Takeuchi in their book 'The Knowledge-Creating Company,' published in 1995.
It is a model that describes how information is transformed into knowledge within organizations. It provides a solid framework for understanding how knowledge is generated and shared within organizations, and these principles have significant implications for how artificial intelligence is being incorporated to improve knowledge management and consequently expand value generation, enhance results, and provide society with precious time to evolve.
The authors argue that the knowledge transformation process occurs in an ascending spiral, passing through four different modes.
They are:
Socialization
In this stage, tacit knowledge is shared among organization members through social interactions, such as informal conversations, shared experiences, and observation.
Tacit knowledge is rooted in personal experiences and is difficult to formalize because it is individual.
As we have seen, through Generative AI, this tacit knowledge begins to be absorbed and becomes available to the company. The risk of 'brain drain' is partially mitigated.
Externalization
Tacit knowledge is transformed into explicit knowledge. This involves expressing and codifying tacit knowledge in forms that can be communicated and shared more broadly, such as documents, models, narratives or workshops.
These two steps of the Knowledge Spiral – Socialization and Externalization – are highly enhanced by AI, with the expansion of the capacity for sharing and formalizing knowledge, given the current technologies of Big Techs, and others, delivering the automation of these processes through interactions with systems using natural language as a facilitator.
Combination
Explicit knowledge is combined with other types of explicit knowledge to create a broader body of knowledge. Combination involves integrating different perspectives and information to create ideas and insights.
In this step of the Knowledge Spiral theory, AI can learn and generate more and more knowledge through machine learning/deep learning.
However, regarding Combination, which involves integrating different forms of explicit knowledge, it is important to recognize that complete automation of this process can be challenging in many cases.
While AI can process data from various sources, effective Combination, in addition to collaboration among employees, often requires human judgment to ensure the relevance and quality of the combined information.
Therefore, total automation of Combination may not be feasible in all scenarios. It will depend on the selected use case for an agent conception.
Internalization
Finally, the combined knowledge is internalized by the organization's members, becoming part of their individual knowledge base. This allows the knowledge to be practically applied in daily tasks and decision-making, and monetized when applicable.
In this stage of the Spiral, data scientists make use of knowledge for value generation, for example, or anyone with access to artificial intelligence tools, previously trained to interact and deliver knowledge, like GPTs (Generative Pre-trained Transformers).
Regarding Internalization, AI can facilitate the incorporation of explicit knowledge into practical experience, providing relevant information and suggestions based on the available knowledge.
Note that the last two stages of SECI, Combination and Internalization, challenge any belief, in my opinion, about AI taking over everything. There are use cases (or business processes) that will always be better handled by humans themselves instead AI agents.
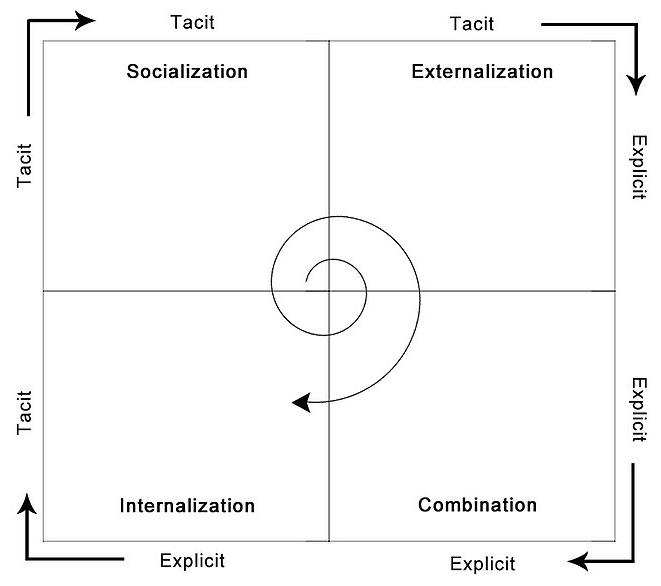
SECI model of knowledge dimensions [http://en.wikipedia.org/wiki/File:SECI_Model.jpg]
The theory emphasizes collaboration as a fundamental element in the creation and transformation of knowledge.
Collaboration is seen by the authors as a means to expand the knowledge base of the organization and promote innovation.
Through Socialization and Externalization, employees of an organization share their individual experiences and collaborate to transform tacit knowledge into explicit knowledge.
As knowledge is Externalized and Combined, new ideas and concepts are generated. This continuous creation of knowledge is seen as a fundamental engine for the growth and adaptation of organizations in a constantly changing environment.
Some examples of collaboration are the digital platforms such as MS Teams, Discord, Slack, Social Networks, product reviews in e-commerce and marketplaces..., where the generated knowledge is stored in an unstructured format in the organizations.
Conclusion
This week I have illustrated how integrating Artificial Intelligence with Knowledge Management, particularly through the Nonaka and Takeuchi Knowledge Spiral, revolutionizes organizational knowledge handling.
AI enhances knowledge transformation from tacit to explicit, fostering innovation and strategic growth. This synergy defines AI's role not as a human replacement, but as a catalyst in making knowledge a more accessible and powerful asset for organizations navigating the complexities of the digital era.
Following my journey revisiting the concepts of eminent thinkers of our era and exploring how Artificial Intelligence can be contrasted and integrated with these principles, next time I will share Peter Drucker's vision for knowledge management, analyze and understand how AI can generate value from actions, which according to him, are responsible for knowledge generation. Without action, there is no knowledge, but only data and information.
Now, tell me here in the comments your opinion.
Do you believe that the Knowledge Spiral theory applies to the management of artificial intelligence in an organization?
Could it be the foundation of a COE (Center of Excellence)? Or are the migrations to AI systems independent and do not require holistic knowledge management?
Do you believe that a digital transformation process from the perspective of implementing artificial intelligence needs to consider prerequisites to be implemented based on knowledge?
Do you think that theories and concepts of knowledge management and its relationship with the company's strategy have any impact on projects for the implementation of an artificial intelligence use case?
Thank you very much to everyone who has followed me so far!

Comments